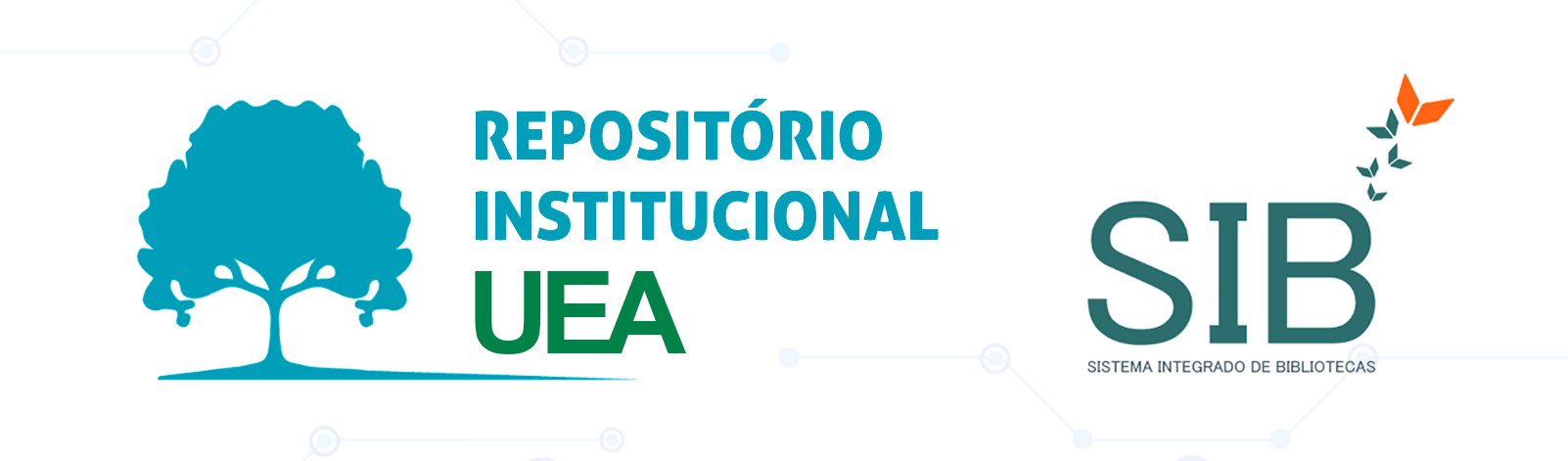
Bitte benutzen Sie diese Kennung, um auf die Ressource zu verweisen:
http://repositorioinstitucional.uea.edu.br//handle/riuea/4483
Titel: | Aplicação de redes neurais artificiais na predição de perfurações durante a soldagem de juntas tubulares em um Chassi Baja |
Sonstige Titel: | Application of artificial neural networks in the prediction of perforations during the welding of tubular joints in a Baja Chassis |
Autor(en): | Guimarães, Pedro Henrique Ferreira |
metadata.dc.contributor.advisor1: | Cruz Neto, Rubelmar Maia de Azevedo |
Stichwörter: | soldagem GMAW;RNA;predição de penetração;DOE;MIG/MAG;Neural networks;Python language;prediction |
Erscheinungsdatum: | 30-Mai-2022 |
Herausgeber: | Universidade do Estado do Amazonas |
metadata.dc.description.resumo: | A qualidade das juntas soldadas em um chassi automobilístico é essencial para garantir a segurança e seu desempenho. A fim de reduzir custos, cumprir prazos e assegurar o máximo de qualidade, o conhecimento sobre parâmetros de soldagem e sua regulagem é fundamental para operações. O objetivo deste estudo é treinar uma rede neural artificial para predição de perfurações em juntas soldadas com o processo GMAW e eletrodo ER70S-6 em tubos de aço SAE 1020 de um chassi Baja SAE. Foram utilizadas como variáveis preditoras o tipo de gás de proteção, diâmetro do arame eletrodo, posição da chave de tensão da fonte e velocidade de alimentação do eletrodo. Dois cordões de solda são soldados em cada corpo de prova, seguindo as configurações determinadas por um DOE (Design of Experiments). Ao final das soldagens, observou-se quais amostras apresentaram perfurações no metal de base. Feitas as observações, foi treinada uma rede Perceptron multicamadas em Python e comparou-se as respostas do modelo aos ensaios. O modelo gerado apresentou uma acurácia de 78% e uma sensibilidade de 76% para dados com quais não foram treinados, revelando o potencial do uso de redes neurais artificiais para predição de perfurações em juntas soldadas. |
Zusammenfassung: | The quality of welded joints in an automobile chassis is essential to ensure safety and performance. In order to reduce cost, meet deadlines and ensure maximum quality, knowledge about adjustment settings and their adjustment is fundamental for operations. The objective of this study is to train an artificial neural network for prediction of burn-through in welded joints using the GMAW process with ER70S-6 electrode in SAE 1020 steel tubes of a Baja SAE chassis. Electrode wire diameter, type of shielding gas, source voltage and feed speed were used as predictor variables. Two weld beads are welded to each specimen, following the configurations determined by a DOE (Design of Experiments). Following the weldings, it was observed which bodies showed burn-through in the base metal. After the observations, a PMC network was trained in Python and the model's responses were compared to the tests. The model presented an accuracy of 78% and a sensitivity of 76% for data, which was not trained, revealing the potential of using artificial neural networks to predict burn-through in welded joints. |
URI: | http://repositorioinstitucional.uea.edu.br//handle/riuea/4483 |
Enthalten in den Sammlungen: | EST - Trabalho de Conclusão de Curso Graduação |
Dateien zu dieser Ressource:
Datei | Beschreibung | Größe | Format | |
---|---|---|---|---|
Aplicação de redes neurais artificiais na predição de perfurações durante a soldagem de juntas tubulares em um Chassi Baja.pdf | 1,98 MB | Adobe PDF | Öffnen/Anzeigen |
Alle Ressourcen in diesem Repository sind urheberrechtlich geschützt, soweit nicht anderweitig angezeigt.